New RPAS Capabilities at Manaaki Whenua
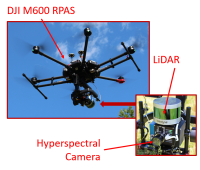
Figure 3. Manaaki Whenua’s new integrated airborne sensing system, comprising a hyperspectral camera and a LiDAR scanning unit mounted together on a DJI M600 hexacopter.
Remotely Piloted Aircraft Systems (RPAS, otherwise known as drones or UAV) have revolutionised our ability to obtain incredibly fine-scale imagery of the land surface with unprecedented ease, affordability, flexibility, and repeatability. RPAS are now widely used in a professional capacity by researchers and land managers to monitor and manage their areas of interest. However, while the technology that allows RPAS to fly and gather imagery has advanced substantially in the last 5 years, the ability to gain actionable information from the detailed data is still in its infancy. Manaaki Whenua has taken a keen interest in RPAS technology, not only to enhance its own science, but also to conduct the research that enables this technology to be used in operations by other management professionals.
The three goals of Manaaki Whenua’s RPAS programme are to: (1) develop and apply specialist methods to answer land managers’ questions; (2) develop and apply RPAS techniques as a research tool to support other science programmes; (3) develop RPAS-based solutions that enable end users to use their own drones to address problems affecting their enterprises. An example of this last goal might be developing a turn-key solution to detect and selectively spray a disease in a high value crop. Over the last several years, we have been developing capability in this area and have recently invested in a set of advanced, research-grade RPAS and sensors.
Our work to date has included capturing RGB (Red-Green-Blue) imagery with standard cameras mounted on RPAS. We have developed methodologies for a range of applications, including characterising riparian strip restoration, mānuka–kānuka distinction at fine scale (based on differences in flowering attributes), and determining sediment losses during stream bank erosion events (Fig. 1). A key tool in developing these methodologies is ‘Structure-from-Motion’ — a digital technique that creates 3D surface models from a set of 2D images.
We have also developed specialist techniques to guide RPAS missions in extreme terrain. This has allowed us to create a digital surface model (DSM) of the dramatic slopes of the Te Mata Peak track, an area where the relief is too variable to rely on the standard RPAS guidance tools. This high-resolution DSM can be further processed to provide spatially explicit guidance for the planning of restoration efforts (Fig. 2).
For many applications, a standard RGB camera becomes limiting when characterising a surface – there are only three spectral channels (red, green, and blue) and each channel is rather wide (i.e. non-specific). A hyperspectral camera contains many more channels and each channel is much narrower (i.e. specific). Moreover, a hyperspectral camera measures light not only in the visible part of the spectrum but also in the near-infrared, a region of wavelengths that contains a great deal of information that help us distinguish different species of plants, diagnose plant health, and estimate soil parameters.
We use an integrated drone/hyperspectral camera system to gather data for 270 spectral channels that span the visible and near infra-red spectrum and provide up to 1-cm resolution (Fig. 3). The system also incorporates a LiDAR sensor that will provide a detailed 3D model, not only of the vegetation surface but also the underlying terrain.
Our new kit also includes advanced thermal imaging sensors for use in mammalian pest research and precision agriculture, and a fixed-wing RPAS with the capability for RGB and multispectral imagery to be obtained at high resolution and accuracy. The extended range of fixed wing RPAS will allow whole farms to be surveyed.
This set of equipment, combined with the capability of the RPAS researchers, will provide flexibility to map multiple attributes of the land surface – spectral, structural, and thermal – at high resolution. Fusion of multiple maps also has powerful potential, for example, digital surface models generated from LiDAR provide key information about the morphology of individual trees. Combining this information with the spectral characteristics of those individuals using a hyperspectral camera opens the door to creating vegetation biodiversity maps at an individual level.
A key part of our RPAS-science workflow will be developing the algorithms that can transform these layers of detailed geospatial data into actionable information that can be used by land managers and researchers. Object recognition and machine learning are key elements of this workflow.
Our RPAS capability will be used to support existing recently funded MBIE research programmes, such as ‘Advanced Remote Sensing Aotearoa’ and ‘Cost-Effective Targeting of Erosion Control to Protect Soil and Water Values’. We are interested in new collaborations with researchers and land managers to develop RPAS pipelines to address important management concerns.