Editorial: Using quantitative models to gain insights in ecology
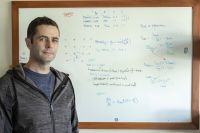
Andrew Gormley
“Mathematics without natural history is sterile, but natural history without mathematics is muddled” − John Maynard Smith
It is probably a fair assumption that people who are interested in the life sciences are not secretly yearning to learn and apply quantitative methods. Indeed, for many professional ecologists, mathematics and statistics were their least favoured subject areas during their training. However, because ecology typically involves trying to make sense of a complex system, we often require quantitative models to help us in that endeavour. A wide variety of quantitative modelling approaches are available, and this issue of Kararehe Kino presents a sample of these methods and how they can be applied to various wildlife management issues.
Some studies require statistical models. These are applied in studies that involve collecting data, where the statistical model is used to analyse that data so that the researcher can interpret the results in order to make reliable inferences about the study. Statistical models are often used in wildlife management to determine the success or otherwise of management. For example, we might collect data on stoat activity in a region before and after stoat control and use a statistical model to provide evidence that any observed change in activity (e.g. a decrease in stoat activity) is a result of the management intervention. Less common, but no less important, is conducting a power analysis before the field study is carried out. A power analysis gives the research team insight into how much field effort they need to undertake to answer their question. If you didn’t find evidence for a change in stoat activity due to control, maybe you didn’t measure it well enough! Cecilia Latham and Dave Latham (Wielding power for pest control) show that conducting a priori power analyses can ensure resources are not wasted on poorly designed studies that would never answer the question of interest.
At other times we require theoretical models. These are often a simplified simulation model of the system being studied to help us understand the system and how its different components interact. These models can be used to tackle complex ecological problems. Pablo Garcia Diaz (Using computer models to understand reinvasion) used a simulation model to provide insight into potential reinvasion by ferrets in a large landscape. His approach illustrates how a simple, yet realistic, model can be used to explore the system process. Furthermore, by exploring how the results of the simulation respond to changes in the input parameter values, such models can help prioritise which field studies are required to better inform the model.
Statistical and theoretical models have the common aim of attempting to provide insight, whether to better understand an ecological process or to assess the success of a management activity. Such models are invaluable to land managers, helping them in their decision-making. For example, our intuition says that if we apply predator control then we will achieve biodiversity benefits. But more nuanced questions, such as how much control we need to carry out, how often, and where to apply it, are more difficult to answer. Models can be a useful tool to guide managers in answering these kinds of questions.
Dean Anderson and colleagues (Modelling supports nimble predator management) used a simulation model to assess the most effective management strategy in order to increase populations of kiwi. Their model forecasts kiwi populations as a result of predator management by accounting for a range of interacting factors such as forest dynamics, and rat and stoat population trajectories.
In a similar vein, Andrew Gormley and Bruce Warburton (TrapSim) constructed a model of predator trapping to help managers decide between the probable effectiveness of various large-scale trapping options. They have also constructed an online, easy-to-use version of a model called TrapSim, which allows managers to explore various scenarios without requiring them to have knowledge of the underlying mode, or training in quantitative methodology.
When trying to eradicate a species, the issue of when managers can declare success is difficult: if the species is not detected, is that because it really has been eradicated or did the field team just not look hard enough? Dean Anderson et al. (article 6) have developed an analytical approach to help decision-makers decide when they can declare successful eradication, and they demonstrate its use on an eradication of Argentine ants on Kawau Island. Their approach is always for multiple surveillance methods to be included and enables managers to understand which of these methods is the most effective.
“Truth is much too complicated to allow anything but approximations” − John von Neumann
Because ecological systems are complex, ecological models are necessarily a simplification of the actual system. Model complexity is a trade-off between simplicity and accuracy. Adding complexity will often improve the realism of a model, but it can make the model difficult to understand. In general, if we have two models with similar predictive power, the simplest one is the most desirable.
This is not to say the simplest model is necessarily the best. For example, the current version of TrapSim mentioned above assumes that all individuals conduct themselves in the same way with respect to home range and how they interact with traps. But if that’s not the case, does it matter? Giorgia Vattiato (How behavioural differences in pests...), a PhD student working with Rachelle Binny, is using a model to understand the potential effects of individual behavioural differences on the efficacy of predator programmes. Theoretical models such as these can help determine whether more complicated effects are worth including in the model, as well as providing managers with valuable information on the potential for the trapping programme to fail as a result of these individual differences.
Modelling is not confined to ecology, and is carried out in many disciplines. Network connectivity is a widely studied field in engineering with important applications to traffic management. Tom Etherington et al. (Prioritising pest control) recently applied network connectivity research to invasive pests to help identify areas that can be targeted to ensure greater control efficiencies. Their work demonstrates how applying methods developed within other disciplines can be used to address ecological questions.
The opposite can also be true. Predator−prey models are commonly used in ecology to make inferences about the dynamics of two interacting species (e.g. fox and rabbit populations). Bruce Warburton et al. (Economic aspects of NZ's wild deer recovery industry) applied this ecological modelling framework to wild deer recovery, where helicopter hunters are the predator and deer are the prey. By accounting for the various financial costs, they provide a framework to easily assess the economic viability of wild deer recovery in New Zealand.
Models can be extended to include more than just ecological components. Decision-makers also have to consider economic and ethical factors, but accounting for these is inherently difficult. Bruce Warburton and Dean Anderson (Ecology, economics and ethics) present a framework that considers the probabilities of success of each of these factors with respect to a wildlife management programme. Modelling frameworks such as this that also account for ethical considerations will become increasingly relevant, especially as wildlife management moves even more into the public arena with national-scale programmes such as Predator Free NZ
“Essentially, all models are wrong, but some are useful” – George Box
One final note: good quantitative models have the potential to help us better understand an ecological system as well as providing managers with a more objective way of making decisions. However, ‘good’ quantitative models rely on the availability of good data to develop and verify the reliability of the model. Quantitative models are a powerful tool, but they only work in conjunction with good information and are not a substitute for data. Garcia et al. (2019) have recently published a paper describing good practices in quantitative modelling for conservation management, and it is highly recommended as further reading.
Andrew Gormley