How do behavioural differences in pests affect their trapability and eradication success?
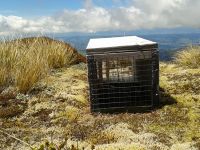
DOC 200 predator trap at Mt Arthur, Kahurangi National Park. Image: Rachelle Binny
New Zealand has a history of successful small mammal eradications from offshore islands. On the mainland, advances in pest-proof fencing technologies have made it possible to eradicate pests over relatively small areas and to protect against reinvasion. Since the announcement of the Predator Free 2050 goal in 2016 there has been increased interest in the development of new tools and strategies to improve the efficiency, effectiveness and scale of pest eradication operations.
Pest managers benefit from models that can make accurate predictions about the size and/or spread of a pest population over time or the outcomes of different management scenarios. For example, models that predict the number of trap nights required for successful eradication under a particular trapping regime can help managers choose an optimal trapping strategy. Such models usually take into account an animal’s movements and use of space, reproduction rate and immigration patterns. What is seldom considered, however, is the possibility that different individuals within a population could exhibit consistent differences in their behaviour. Such differences could have considerable effect on the dynamics of a pest population, and on the effort required for its eradication.
It has been well reported, for example, that animals show varying degrees of boldness when confronted with new objects in their environment. While some animals are quick to approach and interact with unfamiliar objects (e.g. traps or toxic baits), others are more cautious, and a few are highly risk averse. These behavioural differences are a key challenge faced by managers attempting to eradicate – as opposed to suppress – pest populations down to the last individual. Current standard trapping methods often fail when a number of very ‘shy’ individuals are present in the target population because these individuals are unlikely to approach such control devices.
To study this problem and measure the effect of these behavioural differences on control programmes, Giorgia Vattiato, Rachelle Binny, Michael Plank and Alex James have built a model to simulate the evolution of a theoretical population of small mammal pests during a trapping regime. They compared predictions for both a homogeneous population (in which all individuals behave the same (i.e. have the same level of ‘trap shyness’) and a heterogeneous population (in which individuals have different levels of trap shyness) to assess the extent to which behavioural variation matters. Their first simulation results (Figure 1) showed that, as expected, heterogeneous populations (blue line) take much longer to eradicate than homogeneous populations (red line) (more than 350 days versus 135 days, respectively) due to the presence of a few elusive individuals that are extremely difficult to capture.
Another challenge faced by pest managers is how to decide when during an eradication process one can be confident that the target population has been eliminated. Usually this decision is based on the number of ‘quiet nights’ (the time elapsed since a pest was last captured or detected). Giorgia and colleagues used their model to make predictions of this value, once again comparing results for homogeneous and heterogeneous populations (Figure 2). Again, there is a considerable difference between predictions for the two simulated population types, highlighting the importance of taking such behavioural variation into account in models for pest management. For example, in the overly simplistic model where it was assumed all animals were equally likely to interact with a trap (a homogeneous population), after about 80 quiet nights the probability of having successfully eradicated the population was 0.95. In contrast, for a heterogeneous population (with some trap-shy individuals) nearly 250 quiet nights would be needed to achieve the same probability of success.
So, how can these types of models be used to help design more efficient eradication procedures? So far the model has been used to simulate a theoretical pest population, using ecologically relevant but arbitrary parameters (e.g. the rate at which an animal moves through its home range). The next step is to calibrate the model for a particular pest species and landscape using data from a real pest population. The calibrated model could then become a useful tool to help design an effective eradication programme and to predict the time and probability of success for operations.
Even highly trap-shy individuals can be captured using more intensive trapping and baiting procedures or specially designed control devices. However, these methods are typically more expensive and/or labour-intensive and too costly for long-term use. The key to a successful and cheaper eradication programme could therefore lie in choosing the optimal time to switch from a standard control approach, which successfully kills the majority of a population, to a more intensive approach targeting any remaining individuals. The model could help managers identify this switch-point.
For example, as shown in Figure 1, the evolution of population size for the heterogeneous population shows a considerable deceleration after approximately 50 days, which indicates that most of the pests have been captured and that a change to a more intensive control method is necessary to eradicate the remaining, very trap-shy individuals. The approach outlined in Figure 2 could then be used to estimate the number of quiet nights needed for a high probability of eradication success.
The next steps in this work are to improve the model by adding more detailed landscape parameters (e.g. different terrains and vegetation types) to better simulate animals’ movements within their home ranges. Giorgia and her colleagues will also explore the idea of knowledge transmission among individuals of a population and through generations, to investigate the impact that learned behaviours might have on a population control programme.
This work is funded by Te Pūnaha Matatini, the CoRE for complex systems and networks.
Giorgia Vattiato (University of Canterbury)
Rachelle Binny (Manaaki Whenua)